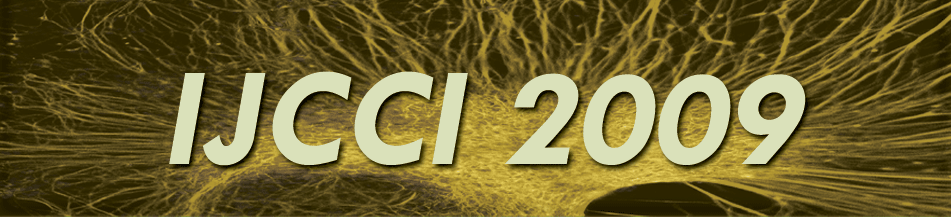 |
International Joint Conference on Computational Intelligence
|
5 - 7 October, 2009 Madeira, Portugal |
|
|
|
|
|
|
|
|
|
|
|
|
IJCCI is a joint conference composed of three concurrent conferences: ICEC, ICFC and ICNC.
The three conferences are always co-located and held in parallel.
Keynote lectures are plenary sessions and can be attended by all IJCCI participants.
Keynote Speakers List:
- Janusz Kacprzyk, Systems Research Institute / Polish Academy of Sciences, Poland
Title: Towards Human /Social Inspired Computation
- Jouko Lampinen, Helsinki University of Technology, Finland
Title: Probabilistic View on Self-organizing Maps and Dimension Reduction
- Leonid Perlovsky, Harvard University, U.S.A.
Title: Language, Cognition and Cultural Evolution
- Qiang Shen, Aberystwyth University, U.K.
Title: Intelligent Systems for Decision Support
- Edward Tsang, University of Essex, U.K.
Title: Evolutionary Computation in Finance Forecasting
|
|
|
|
|
|
Keynote Lecture 1 ICFC |
|
|
|
|
Towards Human /Social Inspired Computation |
|
|
|
|
 |
|
Janusz Kacprzyk
Systems Research Institute / Polish Academy of Sciences
Poland |
|
|
|
|
Brief Bio
Janusz Kacprzyk is Professor of Computer Science at the Systems Research Institute, Polish Academy of Sciences, Professor at WIT - The Warsaw School of Information Technology, and Professor at PIAP – Industrial Institute of Automation and Measurements, allin Warsaw, Poland.. He is Honorary Professor at the Department of Mathematics, Yli Normal University, Shanxi, China, and Visiting Scientist at RIKEN Brain research Insitute in Wako-shi, Japan.. He has been a visiting professor at many universities in the USA, England, Italy, UK and Mexico. He is Academician (Member) of the Polish Academy of Sciences and Foreign Member of the Spanish Royal Academy of Economic and Financial Sciences.
His research interests include intelligent systems, soft computing, fuzzy logic, decision making, decision analysis and decision support, database querying, information retrieval, data analysis, data mining, etc.
He is President of IFSA (International Fuzzy Systems Association), and President of the Polish Society for Operational and Systems Research.
He is Fellow of IEEE and IFSA. He received The 2005 IEEE CIS Fuzzy Pioneer Award for pioneering works on multistage fuzzy control, notably fuzzy dynamic programming, and The Sixth Kaufmann Prize and Gold Medal for pioneering works on the use of fuzzy logic in economy and management.
His publication record is: 5 books, 30 volumes, 300 papers. He is Editor in chief of 3 Springer’s book series, and a co-editor of one Springer book series, is on the editorial boards of ca. 30 journals, and a member of the IPC at more than 200 conferences.
Abstract
Biologically inspired computing paradigms, exemplified by broadly perceived evolutionary computation, immunological, swarm intelligence, ant colony, etc. models have recently attracted much attention in the research community and have shown a considerable potential for solving many complicated real world problems. One can however view them as based on inspirations from primitive living organisms or their colonies. An immediate question is whether it would make sense to devise models based on inspirations from more sophisticated human behavior inspirations, both at the level of individuals and groups. Human individuals or human groups exhibit some sophisticated types of rationality, emotions, and other feelings, and there is usually a leader(s) in social groups.
We follow this path, and to be more specific, we deal with some new computation paradigms in the context of decision making type models. We consider both the decision analytic type and game theoretic types of models, and present some examples of experiments, mainly obtained in the area of behavioral economics and neuroeconomiccs, which clearly suggest a discrepancy between solutions adopted by humans and obtained by using directly the traditional decision analytic and game theoretic models that are in principle based on a greedy utility maximization.
Basically, we emphasize what has been experimentally shown in many cases of real world human decision making that a human being is in general not a deliberate, hence slow, decision maker driven by a greedy and selfish utility maximization, which is a point of departure to traditional formal models, but is rather an emotional, fast decision maker who is often willing to faster arrive at a decision, even if it is “worse”, and – what is maybe more important – whose behavior is often motivated by a willingness to be fair to others, expecting a reciprocal reaction.
We propose some new decision analytic and game theoretic models in which the fairness related elements are explicitly reflected. As an illustration we will show some models of this type which can be used for socio-economic development.
Finally, to find possible explanations for such discrepancies we briefly survey some results of neuroeconomics which is a field about neural mechanisms involved in decision making and their economic relations and connotations. We discuss results of brain investigations which indicate which parts of the brain are activated while performing some decision making related courses of action and provide some explanation about possible causes of discrepancies between the results of formal models and experiments.
|
|
Keynote Lecture 2 ICFC |
|
|
|
|
Probabilistic View on Self-organizing Maps and Dimension Reduction |
|
|
|
|
 |
|
Jouko Lampinen
Helsinki University of Technology
Finland |
|
|
|
|
Brief Bio
Jouko Lampinen received M.Sc. degree in Physics from University of Kuopio, Finland, in 1988 and Ph.D. in Information Technology from Lappeenranta University of Technology, Finland, in 1993.
In 1996 he joined the Laboratory of Computational Engineering, at Helsinki University of Technology, TKK. He is now a professor in computational information technology in the Department of Biomedical Engineering and Computational Science at TKK.
His current research interests include statistical learning, especially Bayesian methods and MCMC techniques with applications in machine vision, health data analysis, and brain imaging.
Abstract
Self-organization is one of the key concepts in development of emergent properties in systems with large number of degrees of freedom. In broad view self-organization can be defined as an unsupervised process that creates structures from data, so that the structures can be characterized by fewer degrees of freedom than the original data. Common applications of self-organization in data-analysis are dimension reduction (i.e. characterizing the high-dimensional data with lower dimensional new variables) and clustering (i.e. grouping similar data points and characterizing the data as terms of the groups).
During recent years probabilistic approach has gained popularity in computational intelligence and data-analysis. Probabilistic models are based on generative models that define the probability density of the data given the model. Compared to descriptive models, the approach yields more versatile tools for analyzing the uncertainty in the results and predicting system outcomes.
In data-analysis the Self-organizing Map, SOM, is one of the most influential self-organization algorithm, with thousands of applications in visualization and clustering of high-dimensional data. The SOM has been defined constructively, via the update rule, and theoretical analysis of the resulting models has turned out difficult. We show how the SOM can be analyzed in probabilistic terms, as a constrained probability density estimate fitted to the data. This approach allows applying statistical model selection and analysis methods to the SOM.
Dimension reduction is closely related to clustering, as the clusters characterize the structure of the data and dimension reduction should preserve the structure. We review and demonstrate various probabilistic methods for dimension reduction suitable for cluster analysis.
|
|
Keynote Lecture 3 ICNC |
|
|
|
|
Language, Cognition and Cultural Evolution |
|
|
|
|
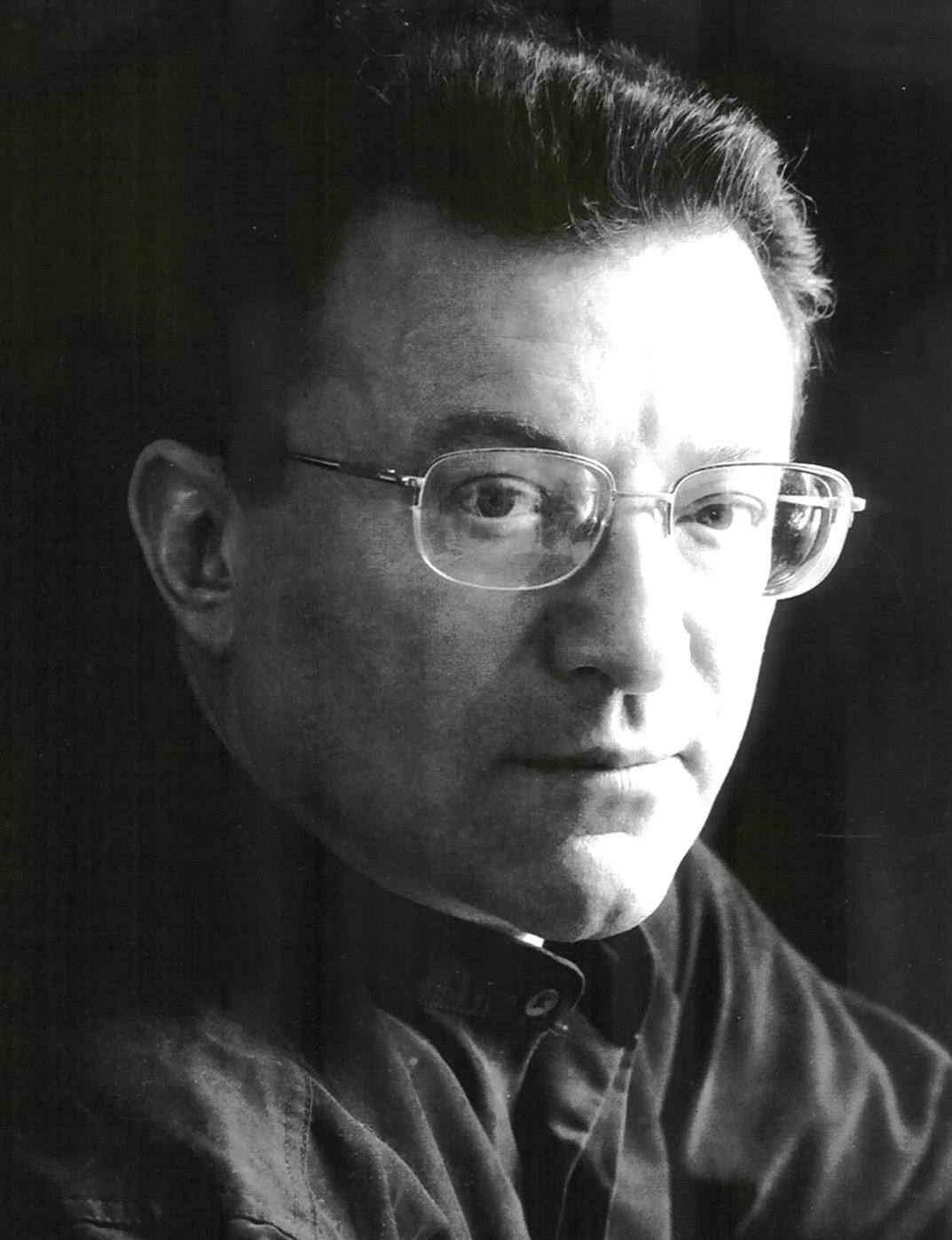 |
|
Leonid Perlovsky
Harvard University
U.S.A. |
|
|
|
|
Brief Bio
Dr. Leonid Perlovsky, Visiting Scholar at Harvard, Principal Research Physicist and Technical Advisor at the Air Force Research Lab; leads programs on socio-cultural modeling, semantic web, cognitive algorithms, prediction, classification, fusion. As Chief Scientist at Nichols Research, a $0.5 B high-tech organization, he led the corporate research in intelligent systems and neural networks; served as professor at Novosibirsk and New York Universities; participated as a principal in startups developing neural tools for language learning, search engines, biotechnology, and financial predictions. His company predicted the market crash following 9/11 a week before the event, detecting ripples from activities of Al Qaeda traders, and later helped SEC looking for perpetrators. He delivered invited keynote plenary talks and tutorial lectures around the globe, published more then 330 papers, 10 book chapters, and 3 books, “Neural Networks and Intellect,” Oxford University Press, 2000 (currently in the 3rd printing) and 2 books with Springer in 2007. Dr. Perlovsky organizes conferences on Computational Intelligence, leads IEEE NNTC and Task Force on “The Mind and Brain,” serves on the Board of Governors of the International Neural Network Society, on Editorial Boards of five journals, including Associate Editor for IEEE Transactions for Neural Networks, and Editor-in-Chief for “Physics of Life Reviews,” which he started jointly with Nobel Laureate I. Prigogine. He received several National and International Awards, including McLucas Award 2007, the highest AF scientific award, and Gabor Award 2007, the highest engineering award from International Neural Network Society.
Abstract
What are the mechanisms of interaction between language and cognition? The talk briefly reviews past mathematical difficulties of modeling the mind and new mathematical techniques of dynamic logic (DL) and neural modeling fields, which overcome these difficulties. DL evolve fuzzy logic into crisp one. Computational and neural mechanisms of concepts, emotions, instincts, imaginations, intuitions are described; they are inseparable from perception and cognition. Engineering applications illustrate orders of magnitude improvement in recognition, data mining, inverse problems, fusion, financial predictions. DL is extended to language and mechanisms of joint operations of language and cognition. It turned out those human abilities could only evolve jointly.
The second part of the talk moves to higher cognitive functions and symbolic abilities. Neural computations explain roles of beautiful, music, sublime in the mind, cognition, and evolution. I relate DL to the knowledge instinct, which drives the mind to understand the world, and argue that instinct is even more important than sex or food. Two mechanisms of the knowledge instinct, differentiation and synthesis determine evolution of cultures, and explain three types of cultural evolutions. The knowledge-acquiring cultures lead to science and technology, but doubt their values; traditional cultures invest limited amount of knowledge with strong emotions, values are certain but knowledge stagnates; multi-cultural societies combine knowledge and values. Different languages lead to different emotionalities.
The brain imaging experiments has confirmed DL and language-cognition interactions. The Nobel Prize in Economics, 2002, is related to the Biblical story of the fall. Neural computations bear on contemporary world, they may improve mutual understanding among peoples around the globe and reduce tensions between cultures.
|
|
Keynote Lecture 4 ICFC |
|
|
|
|
Intelligent Systems for Decision Support |
|
|
|
|
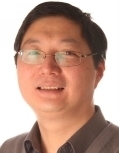 |
|
Qiang Shen
Aberystwyth University
U.K. |
|
|
|
|
Brief Bio
Qiang Shen holds the established Chair in Computer Science and is
Director of Research with the Department of Computer Science at
Aberystwyth University, UK. He is also an Honorary Fellow at the
University of Edinburgh, UK. Prof. Shen's research interests include:
computational intelligence, fuzzy and qualitative modelling, reasoning
under uncertainty, pattern recognition, data mining, and real-world
applications of such techniques for decision support (e.g. crime
detection, consumer profiling, systems monitoring, and medical
diagnosis). He has a total of 27 years of working experience in these
areas. Prof. Shen is also currently an associate editor of two premier IEEE
Transactions (Systems, Man and Cybernetics, and Fuzzy Systems), and an
editorial board member of several other leading international
journals. He has chaired and given keynote lectures at many
international conferences. Prof. Shen has authored 2 research
monographs, and over 230 peer-reviewed papers (a third of which
appeared in world-class journals), including one which received an
Outstanding Transactions Paper Award from IEEE. He has successfully
supervised over 30 PDRAs and PhDs, including a prestigious British
Computer Society Distinguished Dissertation Award winner.
Abstract
Decision support systems play an important role in many application
domains. For instance, in the detection of serious crime, including
terrorist activity, an intelligent system which is capable of
automated modelling and analysis of intelligence data is of great
importance. This will provide useful decision support for intelligence
analysts, offering effective means in the assessment of emerging
scenarios for plausible crimes. Such systems will also facilitate
rapid response to the need of devising and deploying preventive
measures. This talk will describe the important challenges which face
the development of intelligent decision support systems, with a focus
on the problem of crime detection and prevention. It will present some
recent advances in computational intelligence in general, and in fuzzy
systems in particular. These advances contribute to the accomplishment
of tasks essential for intelligence data monitoring (amongst other
applications). The talk will conclude by identifying some significant
potential future developments.
|
|
Keynote Lecture 5 ICEC |
|
|
|
|
Evolutionary Computation in Finance Forecasting |
|
|
|
|
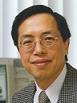 |
|
Edward Tsang
University of Essex
U.K. |
|
|
|
|
Brief Bio
Edward Tsang is a Professor in Computer Science at University of Essex, where he is also a Co-founder of the Centre for Computational Finance and Economic Agents (CCFEA). He was the Founding Chair of IEEE Computational Finance and Economics Technical Committee (CFETC) of which he remains an active member. With background and experience in industry, Edward Tsang has broad interest in business application of artificial intelligence, including computational finance, computational economics and constraint satisfaction. He works with companies including BT, Honda Europe and Sharescope. Main techniques used in his research include heuristic search, optimisation and evolutionary computation.
Abstract
Forecasting is an important activity in finance. Under classical economics, the market cannot be forecast if it is efficient. However, the “efficient market hypothesis” is under serious challenges by recent research. Traditionally, forecasting has been done with in-depth knowledge in finance and the market. Advances in computational intelligence have created opportunities that were never there before. Machine learning, helped by evolutionary computation, can dramatically enhance our ability to forecast. Specialized evolutionary methods can help us to forecast ahead of our competitors and identify scarce opportunities. This talk explains some of the opportunities offered by evolutionary computation and some of the achievements so far. It also explains the underlying technologies and explores the research horizon.
|
|
|
|
|
Copyright © INSTICC
Page updated on
25/01/10
|
|
|
|
|